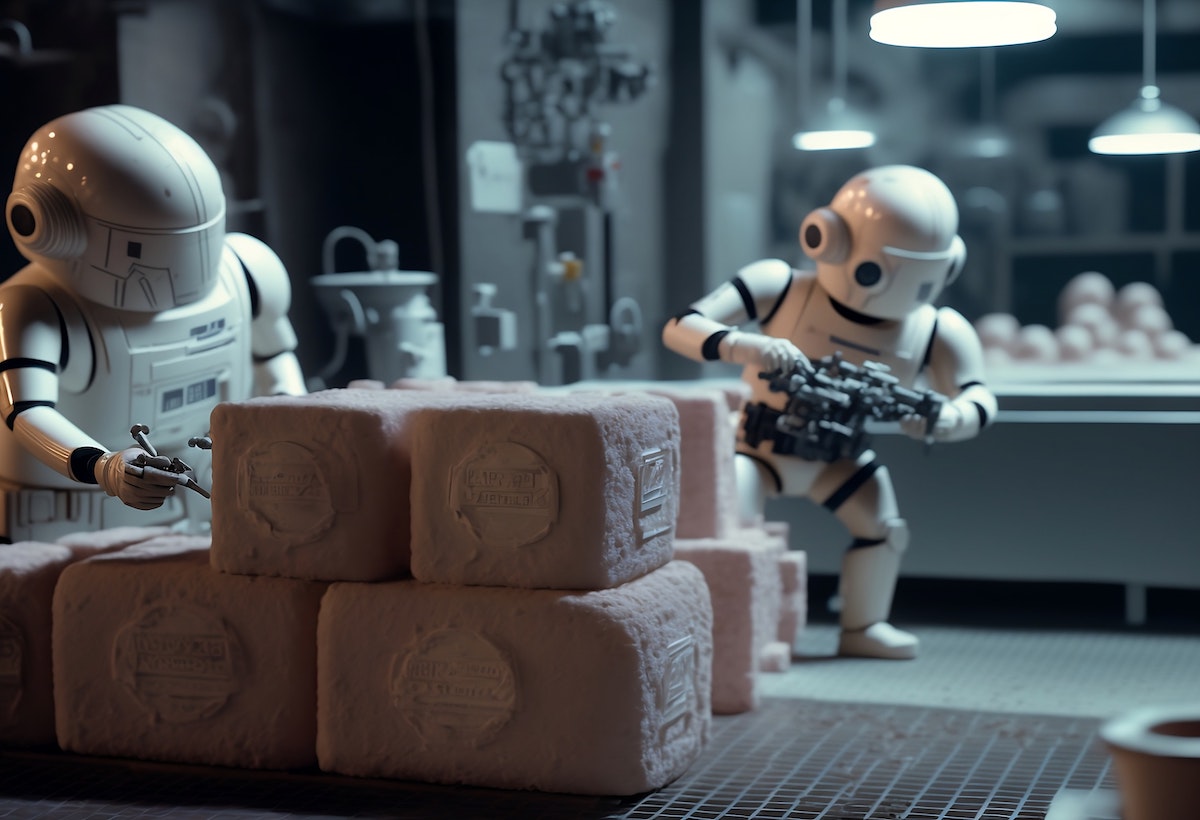
Fine-tuning Large Language Models: Customization for Success in AI Business Development
Introduction
Artificial intelligence (AI) has made significant strides in recent years, particularly in the realm of natural language processing (NLP). Large language models, such as OpenAI’s GPT-3, have emerged as powerful tools for businesses aiming to leverage the capabilities of NLP. While these models possess remarkable abilities straight out of the box, customizing them to cater to specific industries and applications is vital for businesses seeking success in AI-driven development. In this comprehensive article, we will delve into the advantages of fine-tuning large language models, explore methods for customization, and discuss best practices for achieving optimal results.
Section 1: Understanding Large Language Models
Large language models are a type of AI model designed to understand and generate human-like text. They are trained on vast amounts of data from diverse sources, enabling them to learn grammar, vocabulary, and contextual understanding. By utilizing advanced machine learning techniques, these models can perform a wide range of tasks, from generating coherent paragraphs to answering questions and translating languages.
1.1 The rise of large language models
The increasing availability of computational power and big data has fueled the development of large language models. As these models have grown in size and complexity, their capabilities have expanded significantly. The introduction of models like OpenAI’s GPT-3 has demonstrated the potential for AI to generate high-quality, contextually relevant content at an unprecedented scale. This breakthrough has sparked widespread interest in harnessing the power of large language models for various business applications.
1.2 Applications of large language models in business
Large language models have numerous potential applications across a variety of industries. Some of these include:
- Content generation: These models can create articles, marketing materials, and social media posts with minimal human intervention, reducing the time and effort required to produce high-quality content.
- Customer support: Large language models can be employed to assist customer support teams in answering queries and resolving issues, helping to improve response times and customer satisfaction.
- Sentiment analysis: By analyzing text data, these models can gauge sentiment towards a brand, product, or service, providing valuable insights for businesses.
- Translation services: Large language models can quickly and accurately translate text between languages, streamlining communication for international businesses.
Section 2: Benefits of Customizing Large Language Models
Customizing large language models for specific domains and industries offers numerous benefits, some of which are highlighted below.
2.1 Enhanced domain-specific understanding
By fine-tuning a language model to focus on a specific domain or industry, businesses can improve the model’s understanding of specialized terminology, concepts, and context. This customization ensures that the AI produces more accurate and relevant results, enhancing its effectiveness in tasks such as sentiment analysis, customer support, and content generation.
2.2 Improved performance
A fine-tuned model is better equipped to handle specific tasks, resulting in increased efficiency and accuracy. This enhanced performance can lead to faster response times, improved customer satisfaction, and ultimately, increased revenue.
2.3 Tailored user experience
Customization enables businesses to create AI-driven experiences that resonate with their target audience. By training the model on industry-specific data, businesses can ensure that the AI understands and caters to their users’ needs, expectations, and preferences.
2.4 Competitive advantage
A customized large language model can provide businesses with a competitive edge, as it enables them to offer unique and differentiated services. By leveraging the power of AI, businesses can gain insights, streamline operations, and engage customers in novel ways, setting them apart from their competitors.
Section 3: Best Practices for Fine-tuning Large Language Models
To optimize large language models for specific industries and applications, businesses should adhere to the following best practices.
3.1 Define the objective
The first step in fine-tuning a large language model is to clearly define the objective or goal. Identify the tasks that the model will be used for and the desired outcome. This clarity helps to guide the fine-tuning process and measure success.
3.2 Collect domain-specific data
To train a model effectively, it is essential to gather high-quality, domain-specific data. This data should be diverse, representative, and relevant to the industry or problem being addressed. Consider using a combination of public and proprietary data sources to achieve the best results.
3.3 Use transfer learning
Transfer learning is a technique that involves using a pre-trained model as a starting point and fine-tuning it to adapt to a specific domain. This approach saves time and resources compared to training a model from scratch, as the pre-trained model already has a strong foundation in general language understanding.
3.4 Evaluate and iterate
Regularly evaluate the performance of the fine-tuned model using a set of relevant metrics, such as accuracy, precision, recall, and F1 score. This evaluation will help identify areas for improvement and guide further fine-tuning. Iterative fine-tuning ensures that the model continuously adapts to new data and remains effective over time.
3.5 Monitor ethical considerations
AI has the potential to reinforce biases present in training data, which can lead to undesirable outcomes. It is crucial to monitor and address potential ethical issues throughout the fine-tuning process. Implementing fairness and diversity checks, as well as ensuring transparency and explainability, can help mitigate these risks.
Section 4: Case Studies of Successful Large Language Model Customization
To demonstrate the effectiveness of fine-tuning large language models for specific industries, we present two case studies that showcase the tangible benefits of customization.
4.1 Healthcare
A healthcare organization sought to improve its patient communication by leveraging AI-driven chatbots. The organization fine-tuned a large language model to better understand medical terminology, treatment protocols, and patient concerns. As a result, the chatbot was able to provide accurate and personalized information to patients, improving their experience and reducing the burden on healthcare professionals.
4.2 Finance
A financial institution aimed to streamline its customer service operations by implementing an AI-powered virtual assistant. By fine-tuning a large language model to recognize financial terminology, industry regulations, and customer inquiries, the virtual assistant was able to provide timely and accurate support. This customization led to increased customer satisfaction and reduced operational costs.
Section 5: Challenges and Future Directions
Despite the numerous advantages of customizing large language models, there are several challenges and areas for future research.
5.1 Data availability and quality
Access to high-quality, domain-specific data is crucial for successful fine-tuning. However, acquiring such data can be challenging, particularly in niche industries or for proprietary applications. Additionally, ensuring data quality and representativeness is essential to avoid biases and inaccuracies in the resulting AI model.
5.2 Computational resources
Fine-tuning large language models requires significant computational resources. This can be a barrier for small businesses or organizations with limited access to high-performance computing infrastructure. Developing more efficient fine-tuning techniques and making computational resources more accessible will be critical to widespread adoption.
5.3 Ethical concerns
As AI models grow more powerful and ubiquitous, concerns about their ethical implications become increasingly important. Ensuring that large language models are fine-tuned in a way that promotes fairness, diversity, and transparency is essential to maintain trust and avoid unintended consequences.
Conclusion
Fine-tuning large language models for specific domains is an essential step for businesses looking to harness the power of AI for their development. Customizing these models ensures that they are effective, efficient, and tailored to the unique needs